Development of machine learning prototypes for sports and custom wearables.
The design and development of machine learning prototypes should be seen as a complex process where advanced mathematical models, technology, electronics, and, above all, the expertise of industry specialists in the targeted innovation sector coexist.
No es posible desarrollar un prototipo o producto innovador con machine learning, capacidad de diagnosticar de forma inteligente y brindar predicciones basadas en modelos matemáticos, si no se ha entrenado correctamente con un patrón, que además, debe estar condicionado por expertos en la materia. En el presente ejemplo de prototipo machine learning <Baseball Analyticts» podrán analizar los motivos de estas afirmaciones.
Baseball analytics is just one of the examples of prototypes based on Machine Learning technology, which are increasingly common in our technology product design laboratory. The truth is that our main clients for custom IoT product development, as well as custom wearable elements, are sports companies looking to make a difference in the market through intelligent prototypes.
In this prototype example, "Baseball Analytics", the initial objective of the inventors was:
To achieve an innovative and affordable product that provides a fundamental quantitative analysis of batters' swings in different scenarios, allowing for the study of movements, mechanical movement metrics, and delivering guidance to correct poor practices in their batting training sessions.
This is a common objective in IoT product examples with Machine Learning capabilities that we have developed at Let's Prototype for other sports, specifically racket sports such as Tennis and Padel.
Building on our experience designing custom wearable products, we proposed the development of a bracelet capable of managing a set of sensors that provide highly relevant and precise insights into the mechanical movement performed. In addition to the data collected by the Wearable IoT device, other personal, physical, weight-related data, etc., was also gathered through a custom-developed app, which in turn allows for a complete pattern of athletes' behavior in different scenarios.
Once the mathematical model for the machine learning system, developed exclusively for this prototype, is calibrated, the data of correct movement types are parameterized, as well as the ability to compare descriptive data of the batter's mechanical movements with data from batters who, with very similar physical characteristics, achieve highly relevant results in baseball.
In this way, on the mobile app, users of Baseball Analytics and their coaches can graphically see the differences and percentage of similarity between their mechanical movements and those of professional players with highly refined batting techniques and results.
Calculate the Value of Your Patent
Are you ready to find out the estimated value of your Patent or Utility Model?
"Developing high-quality machine learning prototypes requires a highly skilled team with expertise in Mathematics, Electronics, Industrial Design, Mechanical Engineering, Software Programming, and Telecommunications. Attempting to create a machine learning prototype without these professionals will only result in an underperforming proposal with limited chances of success."
Development of products with machine learning and IoT solutions to enhance sports.
As you already know, when we talk about IoT devices, we refer to the invention's ability to connect to the cloud and transmit collected data from the field via a telecommunications system. This way, with reliable data on a server, multiple functions can be developed that add value for users. For example, in this case, the ability of the smart bracelet prototype Baseball Analytics to gather reliable data and send it to the cloud makes it possible to establish a comparison through a machine learning system. This system is based on pre-recorded movements that correspond to highly refined and correct models, enabling real-time feedback or information that provides substantial value to athletes and their coaches.
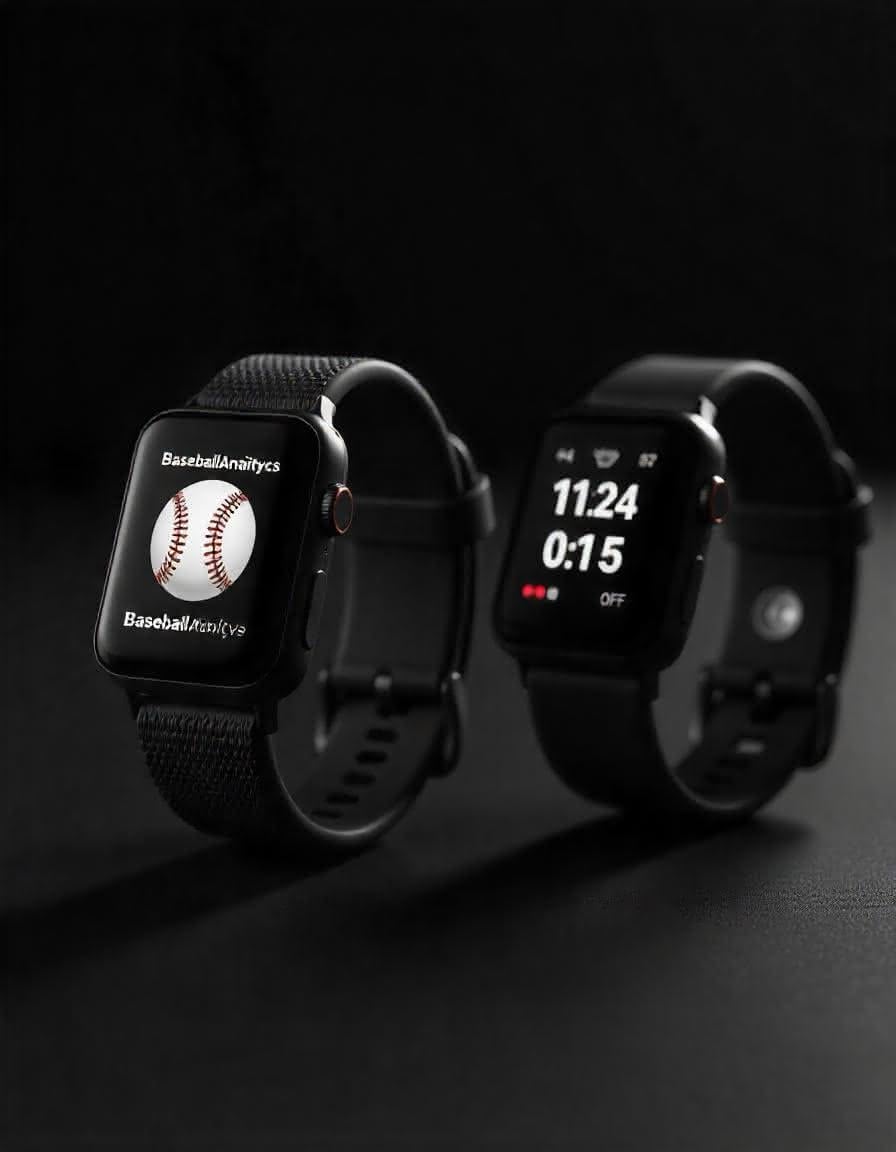
5 Key Tips for the Machine Learning Stage in Prototyping.
Multimodal Data for Machine Learning.
In IoT projects and Machine Learning systems for sports, or other sectors where Machine Learning is driving a true revolution, a prior study of multimodal data that can enhance the Machine Learning model results is essential.
By multimodal data, we refer to data coming from different sources, whether linked to the same wearable device or not, but considered within the same hardware architecture. For example, in this specific case of Baseball Analytics, it was determined that physical data requested through the app, as well as other environmental data captured by the wearable prototype itself, is essential to achieve a more accurate behavior analysis.
We want to bring your ideas to life
CATALOG OF
PROTOTYPES
You can now access over 30 examples of prototypes designed and manufactured in our lab
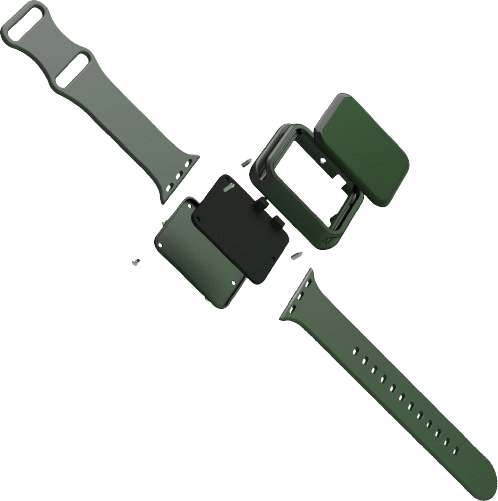
Dynamic Machine Learning.
The machine learning systems that our clients typically request for prototype development are based on basic mathematical models that allow for the capture of critical data for a specific practice, which can then be compared with model or reference data to perform comparative diagnostics. These diagnostics can also take into account other factors influencing the practice, as previously explained in the definition of multimodal data for machine learning.
Some clients of our design laboratory and smart wearable product development request to go a step further with the design and development of machine learning models, as has been the case with Baseball Analytics.
In this case, the client required that the Machine Learning model be capable of adapting to each user based on their specific needs and diagnostics performed during practice with the use of the prototype. For this challenge, which we define as a dynamic Machine Learning model, we developed Deep Learning models based on systematic neural networks, making it feasible to use RNN.
Design and Development of Predictive Machine Learning Models.
In addition to the comparative data analysis system, which was achieved with the development of the Machine Learning model for sports, tailored in this case for the world of baseball, it was possible to establish a predictive analysis using the already collected data. Based on certain critical parameters analyzed in the movement, a relationship was identified that allows for the detection of moments when athletes are more or less focused, predicting the ideal times for them to reach peak performance. This way, coaches can not only work on correcting their athletes' movements but also rely on certain athletes depending on a set of conditions, enabling them to win more games.
Machine Learning with Real-Time Results.
One of the most relevant tips we have identified in the development of prototypes with Machine Learning, or those that rely on this type of data analysis models, is the ability of training models to respond immediately, a crucial factor in most Machine Learning projects for sports that we have designed and manufactured as a prototype at Let's Prototype. This preliminary experimental analysis will be conducted beforehand to optimize the latency of the appropriate hardware elements for the prototype before beginning the data collection process for the design of the Machine Learning model</b
Comparison Logic with Advanced Machine Learning Methodologies.
Typically, developers of Machine Learning projects view the data analysis system and diagnostic comparisons as a whole. After gaining experience in the development of predictive models with custom mathematical models for different sports prototypes, it has been found that breaking down the analyses into parts is a technique that yields excellent results. In this specific prototype example, the analysis captured by the smart bracelet was divided into three fundamental phases: preparation, contact, and follow-through. This phased comparative analysis is much more specific and adds even more value to users. In this case, with the Machine Learning prototype, coaches can not only make full comparisons of a mechanical movement but also correct it in a partial and progressive manner, leading to more efficient, goal-focused training with results that are verifiable more immediately. This is ultimately a key motivational tool for athletes.
As a general tip, it is important that, from the perspective of an inventor or a company’s innovation department, you understand that before proposing a Machine Learning model for sports, a set of decisions must be made collaboratively and only after conducting some experiments. This research phase is essential to develop Machine Learning products that provide real value and truly differentiate the prototype from the competition.
In this prototype example, our client's innovation department had a very clear vision of the possibility of analyzing movement through data taken from the batter's arms directly on the movimiento mecánico, pero, por ejemplo, no tenía en cuenta que:
Capturing and cleaning data by stages of the actual movement increased the reliability of the mathematical model by more than 80%. These figures were demonstrated with the use of the first version of a functional prototype.
During the design process of a Machine Learning project, non-technical discussions with subject matter experts are essential. From this type of analysis, quantifiable parameters are often defined that can complement the analysis and allow for a higher level of reliability, as well as the development of predictive systems, which add substantial value to this type of prototype.
The time to bring your ideas to life is now. We accompany you throughout the entire process: from idea to product.
San Juan Ingenieros, S. L, is the owner of the domain www.letsprototype.com, and in accordance with the General Data Protection Regulation (EU 1679/2016), we will process your data exclusively to handle your information request. You have the right to rectify or request the deletion of your data at any time via hello@letsprototype.com.